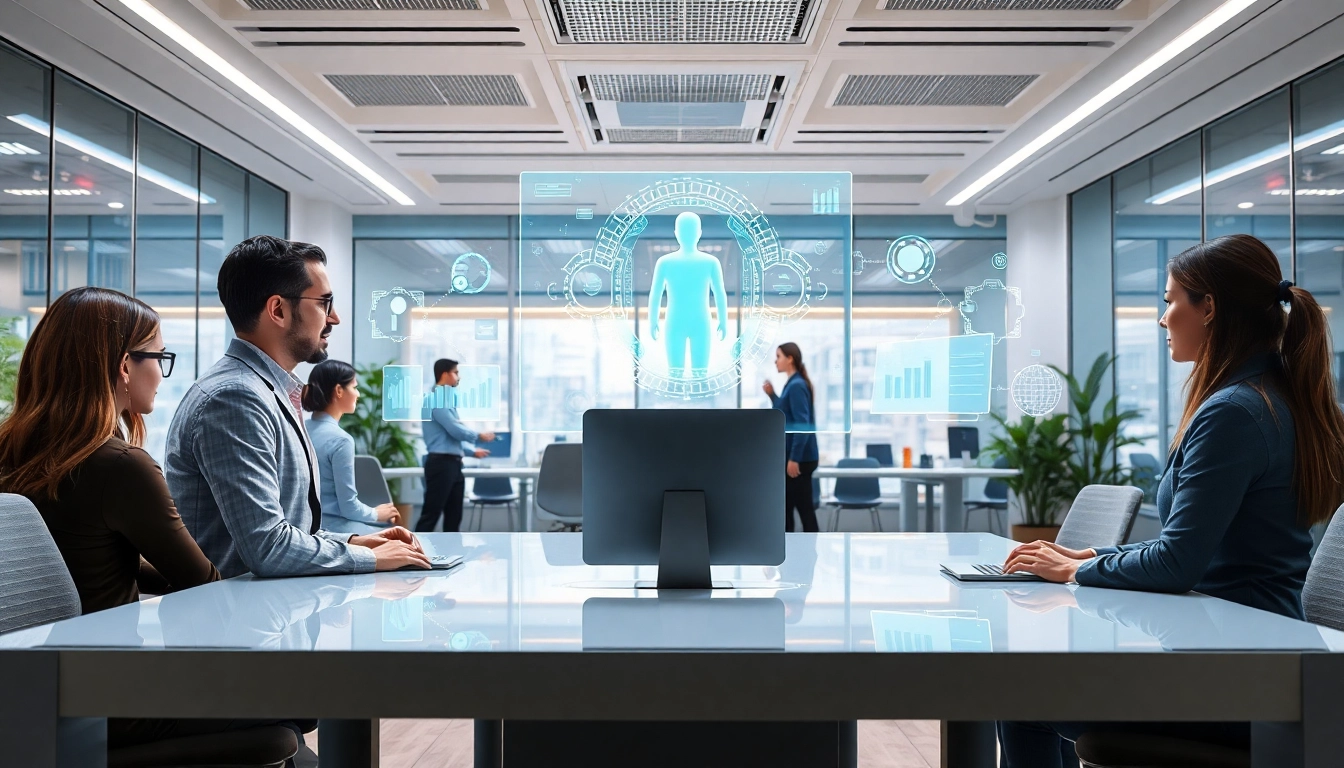
Understanding AI Sales Agents
What are AI Sales Agents?
AI sales agents, often referred to as AI SDRs (Sales Development Representatives), are advanced software applications designed to automate various aspects of the sales process. They leverage technologies such as machine learning, natural language processing (NLP), and data analytics to efficiently perform tasks that traditionally required human intervention. These tasks include lead generation, personalized outreach, scheduling meetings, and providing customer support. By employing an AI sales agent, organizations can significantly enhance their sales efficiency and improve customer engagement.
How AI Sales Agents Function
The functionality of AI sales agents is largely grounded in their ability to analyze vast amounts of sales and customer data. They function by employing algorithms that learn from historical interactions and outcomes, thereby optimizing their sales tactics over time. Most AI sales agents combine several capabilities:
- Data Analysis: They evaluate existing data to identify potential leads and lucrative opportunities.
- Natural Language Processing: This enables them to understand customer inquiries and respond appropriately, often through chatbots.
- Automation: AI systems can automate repetitive tasks, such as follow-up emails or data entry, freeing sales teams to focus on high-impact activities.
Key Benefits of Implementing AI Sales Agents
AI sales agents deliver a multitude of benefits to organizations seeking to optimize their sales processes:
- Increased Efficiency: By automating routine tasks, AI agents allow human sales representatives to concentrate on building relationships and closing deals.
- 24/7 Availability: They can engage with customers at any time, providing immediate responses and support, thus enhancing customer satisfaction.
- Data-Driven Insights: AI sales agents analyze customer behaviors and preferences, offering valuable insights that can inform sales strategies.
- Cost Savings: Reducing the need for a large sales team can lead to significant cost reductions while maintaining or improving productivity.
- Scalability: AI applications can easily scale to accommodate increased workloads, facilitating growth without the need for extensive additional resources.
Types of AI Sales Agents
Chatbots and Virtual Assistants
Chatbots and virtual assistants represent one of the most common implementations of AI sales agents. These tools are designed to handle customer inquiries through text or voice interactions. They can manage everything from simple FAQs to more complex queries, providing personalized responses based on user input. For example, a virtual assistant might guide a customer through a purchasing process, suggest products, or assist in troubleshooting a problem. This instant accessibility greatly enhances customer engagement and satisfaction.
Lead Generation Technologies
Lead generation is crucial for any sales-oriented business, and AI sales agents excel in this area. By harnessing machine learning algorithms, these agents identify and qualify leads through data scraping from various online sources, such as social media, company websites, and public databases. Some systems can even assess the likelihood of a lead converting into a sale based on prior interactions or similar customer profiles. Consequently, businesses can prioritize the most promising leads, increasing their overall conversion rates.
Sales Automation Tools
Sales automation tools streamline the operational side of sales processes, which can include managing teams, tracking customer interactions, and analyzing sales performance metrics. AI-powered sales tools ensure that these processes run smoothly and effectively. They can schedule meetings, follow up with customers, and send out personalized emails without human intervention, allowing sales representatives to focus more on direct engagement and strategy development.
Best Practices for Integrating AI Sales Agents
Assessing Business Needs
Before implementing an AI sales agent, organizations should thoroughly assess their specific needs. This involves understanding the sales process, identifying bottlenecks, and determining which tasks could benefit most from automation. Conducting surveys or interviews with sales teams can yield insights into existing challenges within the sales cycle, guiding the selection of appropriate AI tools.
Choosing the Right AI Solutions
The market for AI solutions is vast and diverse, featuring products tailored to various aspects of the sales process. Organizations should evaluate multiple options, considering factors such as ease of integration with existing systems, scalability, and user-friendliness. Also, organizations should assess the quality and reputation of customer support, as this will impact their experience during implementation and beyond.
Monitoring Performance Metrics
Once AI sales agents are deployed, continuous monitoring is essential. Organizations should establish key performance indicators (KPIs) to track the effectiveness of AI tools. Metrics typically include lead conversion rates, customer engagement levels, and time saved on manual tasks. Regularly reviewing these metrics allows for timely adjustments and optimization of the sales process.
Examples of Successful AI Sales Agents
Case Study: Automation in Real Estate Sales
In the real estate industry, the adoption of AI sales agents has led to significant improvements. An exemplary case is an agency that utilized a chatbot to engage and educate potential homebuyers. The AI agent was programmed to provide property information, schedule tours, and even follow up after visits. By automating these initial customer interactions, the agency was able to increase conversion rates by 30%, as agents could focus their time on interested buyers.
Case Study: Streamlining B2B Sales Processes
In a B2B context, a software company implemented an AI sales agent to manage lead generation and outreach. By automating the discovery of new leads and delivering personalized emails, the AI agent diversified the company’s prospecting resources. This resulted in a 50% increase in qualified leads generated over six months, fundamentally shifting their sales trajectory and enhancing overall revenue.
Case Study: Enhancing Customer Support with AI
AI sales agents are also reshaping customer support in software companies. One such company integrated a virtual assistant to field common inquiries and troubleshoot common issues. By offloading these routine tasks to AI, human representatives were liberated to handle more complex cases. This dual-agent approach led to improved customer satisfaction rates, with many customers expressing appreciation for the immediate assistance provided by the AI agent.
Future Trends in AI Sales Agent Technology
Impact of Advanced Machine Learning
As machine learning continues to evolve, AI sales agents will become even more sophisticated. Enhanced learning algorithms will enable these systems to adapt in real-time, adjusting their strategies based on emerging trends and changes in customer behavior. This adaptation could result in more accurate predictions regarding lead conversion and customer preferences, thus sharpening the effectiveness of sales teams.
Adoption of Natural Language Processing
The capabilities of natural language processing (NLP) are expanding rapidly. Future AI sales agents will likely be able to conduct conversations indistinguishable from those held by human sales representatives. With improvements in sentiment analysis, they will also gauge customer feelings and sentiments during interactions, allowing for more tailored responses that can greatly enhance the customer experience.
Shifts in Customer Expectations
As AI technology advances, so too will customer expectations. Individuals will increasingly demand fast, personalized interactions akin to those provided by AI sales agents. Businesses that integrate these technologies will benefit from heightened customer loyalty and engagement, driving better sales outcomes. Consequently, organizations that delay adopting AI solutions risk falling behind as customer service benchmarks evolve.