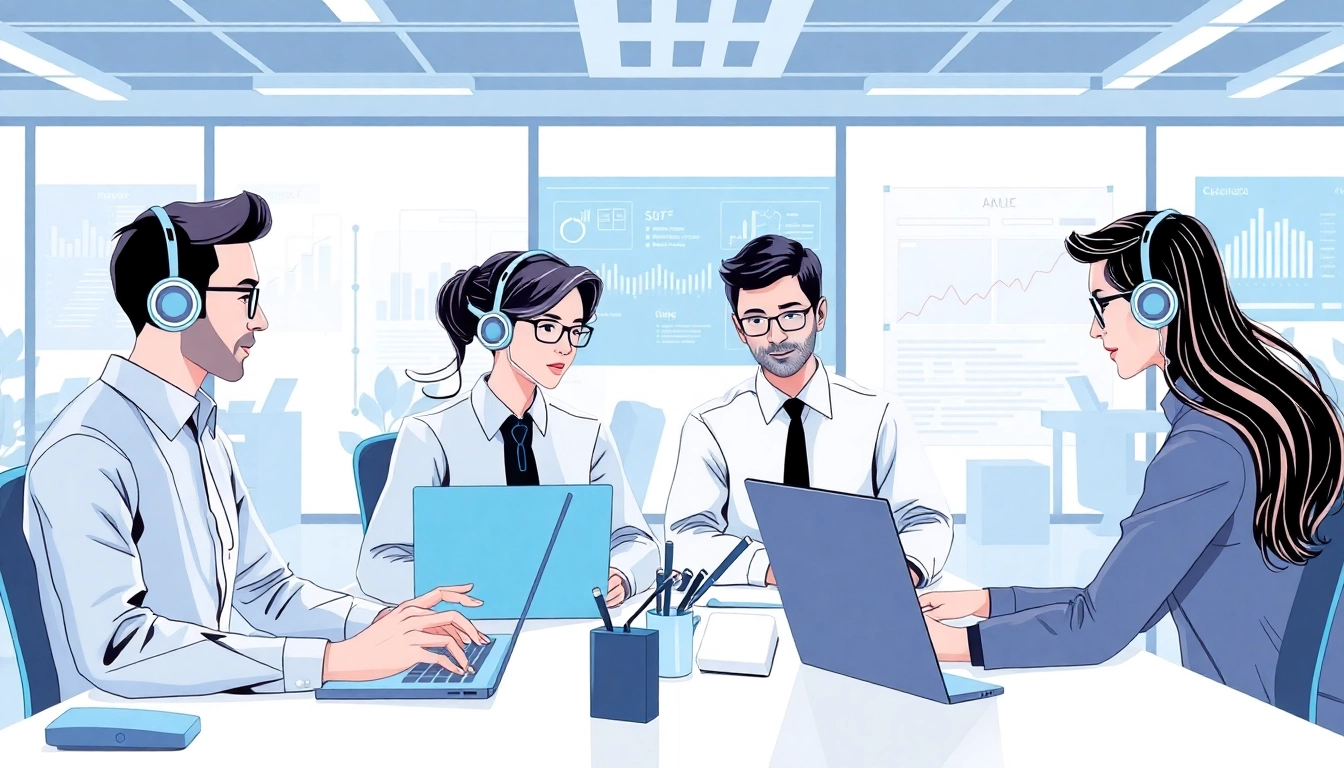
Introduction to AI Agents
As the digital landscape continues to evolve, AI agents have emerged as powerful tools for automating tasks and enhancing productivity. These software systems leverage artificial intelligence to pursue specific goals and complete tasks on behalf of users or other systems. By demonstrating abilities such as reasoning, planning, and memory, AI agents are becoming increasingly autonomous in their operations. This article delves into the intricacies of AI agents, exploring their definitions, historical context, applications, types, benefits, challenges, and future prospects.
What Are AI Agents?
AI agents are intelligent software programs designed to autonomously perform actions or make decisions based on data interpretation and user-defined goals. They function through various layers of complexity, ranging from simple task automation to advanced reasoning and adaptive learning. The autonomy of these systems allows them to operate without continual human intervention, dramatically altering the way businesses and individuals approach tasks.
Historical Context and Evolution
The concept of AI agents is not new; it has its roots in early artificial intelligence research dating back to the 1950s. Initially, the focus was on defining rules and algorithms to simulate human-like decision-making processes. However, advancements in machine learning, natural language processing, and neural networks have exponentially increased the capabilities of AI agents over the decades. Modern AI agents are not just rule-based systems; they incorporate complex algorithms that enable learning from historical data and real-time inputs, adapting their behavior for improved outcomes.
Applications of AI Agents in Real Life
AI agents are utilized across various sectors, showcasing their versatility and impact. Some notable applications include:
- Customer Support: AI-powered chatbots and virtual assistants handle customer inquiries, providing instant support and freeing human agents for more complex issues.
- Finance: AI agents assist in fraud detection, algorithmic trading, and personal finance management, using pattern recognition to analyze vast datasets swiftly.
- Healthcare: In medical diagnosis and patient monitoring, AI agents analyze patient data to recommend treatment plans or alert medical staff to any concerns.
- Manufacturing: In industrial settings, AI agents optimize production processes, monitor equipment performance, and predict maintenance needs to reduce downtime.
Types of AI Agents
Simple Reflex Agents
Simple reflex agents operate on a condition-action rule: if a certain condition is met, they perform a specific action. These agents are reactive and lack memory or learning capabilities, functioning purely on the current input. While they are limited in scope, they can efficiently handle basic tasks, such as controlling light sensors that activate streetlights based on changes in ambient light.
Model-Based Reflex Agents
To overcome the limitations of simple reflex agents, model-based reflex agents maintain an internal model of the world, allowing them to evaluate the current situation based on past experiences. This additional complexity enables them to handle partial information and make informed decisions. For instance, a thermostat can adjust the heating based on both the current temperature and past fluctuations, creating an optimal environment effectively.
Learning and Goal-Based Agents
Learning agents possess the capability to improve their performance over time through experience. They use machine learning techniques to analyze data, learn from outcomes, and refine their actions. On the other hand, goal-based agents prioritize objectives; they navigate through various potential actions to determine the most effective route toward achieving a specific goal. Examples include search engines that learn to deliver more relevant results over time and recommendation systems that tailor suggestions based on user behavior.
Benefits of Using AI Agents
Increased Efficiency and Productivity
AI agents significantly enhance efficiency and productivity by automating repetitive tasks, allowing humans to focus on higher-order problem-solving and creative activities. For instance, in marketing, AI agents can analyze engagement data, optimize ad placements, and even tailor messaging to different audience segments without human intervention. This streamlining of workflows reduces operational costs and accelerates project timelines.
Cost Reduction Techniques
The implementation of AI agents leads to substantial cost reductions across various business operations. By minimizing the need for human labor in routine tasks, organizations can allocate resources more effectively, reducing payroll expenses in the long run. Furthermore, AI agents can help identify inefficiencies in processes, leading to further financial savings through optimized resource allocation and waste reduction.
Enhanced Decision-Making Capabilities
AI agents provide data-driven insights that bolster decision-making processes. With their ability to analyze vast amounts of data quickly and accurately, these agents can uncover patterns that might be missed by human analysis. Businesses leveraging AI agents can make informed decisions based on predictive analytics, market trends, and consumer behavior insights, resulting in improved strategic planning and execution.
Challenges with AI Agents
Data Privacy and Ethical Concerns
With great power comes great responsibility. The use of AI agents raises significant data privacy and ethical concerns. As these systems process personal and sensitive data, companies must navigate strict regulations such as GDPR to ensure compliance. Furthermore, the potential for bias in AI algorithms can lead to unethical decision-making, necessitating a commitment to responsible AI practices that prioritize fairness and transparency.
Integration with Existing Systems
Integrating AI agents into existing infrastructure can pose technical challenges. Organizations often face compatibility issues between AI systems and legacy software or databases, hindering optimal functionality. To address this, businesses should invest in proper integration strategies, ensuring that AI agents can seamlessly connect with current systems to maximize their capabilities.
User Trust and Acceptance
For AI agents to be successfully adopted, users must trust their capabilities. The opacity of AI operations can create skepticism, leading to resistance in utilizing these solutions. Organizations need to prioritize user education, transparency in how AI agents function, and showcasing their benefits to build trust and acceptance among users.
The Future of AI Agents
Trends to Watch
The landscape of AI agents is rapidly evolving. Key trends to watch include the increasing integration of AI agents in everyday applications, advancements in natural language processing for better human-agent interactions, and the emergence of ethical AI frameworks that govern AI development and usage. As businesses strive for digital transformation, AI agents will be at the forefront, driving innovation and efficiency.
Emerging Technologies Enhancing AI Agents
Technological innovations such as deep learning, edge computing, and quantum computing have the potential to further enhance AI agents. These advancements will expand their capabilities, making them more efficient in data processing and decision-making while enabling real-time responses. For instance, edge computing allows AI agents to process data closer to its source, significantly improving response times and reducing latency.
Preparing for a Workforce with AI Agents
As AI agents become more prevalent in the workplace, organizations must prepare their workforce for this transformation. Upskilling employees in AI literacy and collaboration will be essential for ensuring smooth human-AI interactions. Moreover, organizations should focus on creating a culture of adaptability and continuous learning, ready to embrace change and leverage AI agents to their fullest potential.